Unlocking Revenue Potential with Customer Lifetime Value (CLV) Prediction
- Steven Tedjamulia
- Sep 23, 2024
- 5 min read
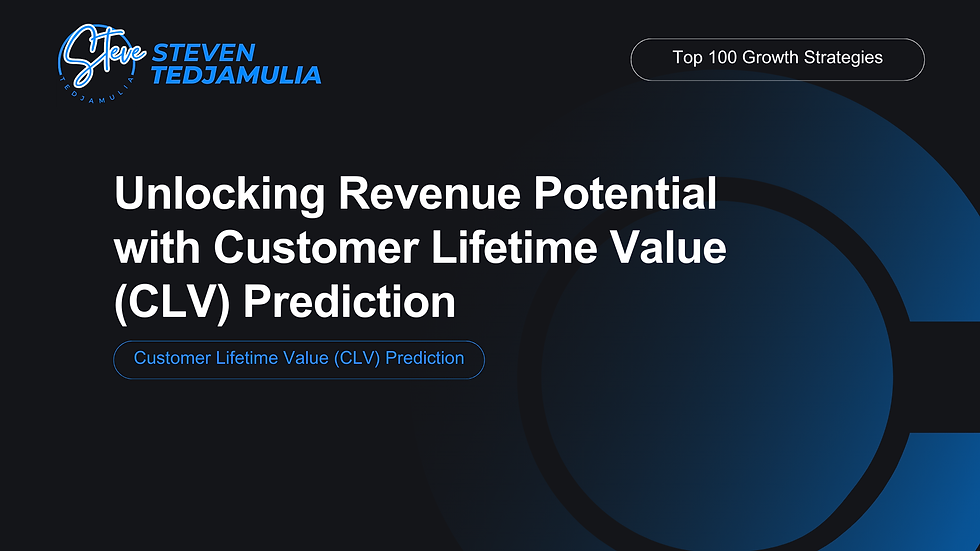
In today’s competitive landscape, businesses must prioritize their most valuable customers to optimize resources and increase profitability. One of the most powerful ways to achieve this is through Customer Lifetime Value (CLV) prediction. By leveraging historical purchase data, customer behavior insights, and advanced AI tools like DataRobot, companies can identify and prioritize high-value customers. This strategy allows for personalized marketing, improved retention, and overall enhanced customer engagement. Whether you're a billion-dollar enterprise or a $10 million business, predictive CLV modeling can provide a significant return on investment (ROI) by enabling smarter, data-driven decisions.
This guide walks you through the essential steps for implementing CLV prediction, the tools and resources required, and the expected ROI for both large and smaller companies. We will also explore the importance of visualizing this data on a dashboard, allowing real-time monitoring of key metrics. CLV prediction is not only a strategic advantage but also a powerful way to streamline customer engagement strategies and boost profitability.
Summary of Steps and ROI
Data Collection and Preparation: Gather purchase history, frequency, average order value, and loyalty program data from CRM systems.
Time: 1-2 weeks
Resources: CRM, SQL, Tableau, data team
Expected ROI: Initial foundation for data model accuracy
Model Building Using AI Tools: Use platforms like DataRobot to build predictive CLV models.
Time: 2-4 weeks
Resources: AI platform (DataRobot), data scientists, data engineers
Expected ROI: Predict high-value customers and allocate resources accordingly (up to 15-20% increase in revenue)
Dashboard Implementation: Visualize CLV predictions through dashboards using tools like Tableau.
Time: 1-2 weeks
Resources: Tableau, data visualization experts
Expected ROI: Faster decision-making, improved campaign targeting (ROI uplift by 10-15%)
Optimization and Continuous Improvement: Refine models and strategies based on performance data and customer feedback.
Time: Ongoing
Resources: Data science team, CRM, optimization tools
Expected ROI: Continuous improvement of CLV models (long-term ROI gains by 20-30%)
Why Customer Lifetime Value (CLV) Prediction Is a Top Use Case
CLV prediction stands out as a critical use case because it directly influences resource allocation, marketing spend, and customer relationship management. By focusing on the most valuable customers, businesses can prioritize their engagement efforts, improve retention, and boost overall profitability. For large enterprises with billions in revenue, this can mean millions of dollars in potential gains by identifying which customers offer the highest returns over time. For smaller businesses, this strategy helps ensure that marketing dollars are spent more effectively and that high-value customers are given the personalized experience they expect.
Predicting CLV enables companies to:
Segment customers more effectively based on predicted future value.
Enhance customer loyalty programs by offering tailored rewards and offers to those most likely to contribute to long-term revenue.
Reduce churn by identifying at-risk customers before they stop purchasing.
Optimize marketing spend by directing resources towards high-ROI segments.
Steps to Implement CLV Prediction
1. Data Collection and Preparation
The foundation of any successful CLV prediction project is high-quality data. For large companies, this typically means accessing vast datasets stored in CRM systems, including:
Purchase history: Transaction records for all customers.
Frequency of purchases: How often customers make purchases within a given time frame.
Average order value (AOV): The mean dollar amount spent per order.
Loyalty data: Any information related to customer engagement in loyalty programs, such as points earned or rewards redeemed.
For smaller companies, the data may be less voluminous but still valuable. The focus should be on accurately tracking the most relevant metrics over time to predict future behavior.
2. Model Building Using AI Tools
Once the data is collected, AI platforms like DataRobot come into play. These platforms can automate much of the model-building process, selecting the best machine learning algorithms and tuning them for maximum accuracy. The goal here is to predict a customer's lifetime value based on historical data and behavior patterns.
Large companies: With extensive datasets, models may take longer to train but can offer more granular insights into various customer segments. The company’s data science team will collaborate closely with AI platforms to fine-tune the models.
Smaller companies: Less data may mean faster training times, but the insights are just as valuable, particularly when marketing resources are limited. Smaller businesses can rely on pre-built models offered by platforms like DataRobot to save time.
3. Dashboard Implementation
Once CLV predictions are in place, it's essential to visualize these insights on a dashboard. Tools like Tableau can be used to create real-time dashboards that display critical metrics, including:
Current and predicted CLV for each customer segment.
Purchase frequency and trends over time.
Churn risk predictions.
Campaign performance related to high-value customers.
These dashboards should be accessible to marketing, sales, and customer success teams, allowing them to make data-driven decisions and tailor engagement strategies accordingly.
4. Optimization and Continuous Improvement
After the initial deployment of the CLV prediction model, continuous monitoring and improvement are crucial. This involves refining the model as new data comes in and testing different engagement strategies to optimize the customer journey.
Large companies: Continuous improvement may involve integrating additional datasets, such as social media engagement or customer service interactions, to enhance predictions.
Smaller companies: Even without large datasets, ongoing feedback loops can help fine-tune the model and improve marketing strategies, especially as new data is collected.
Predictive Returns for Large and Small Companies
For large companies making billions in revenue, CLV prediction can lead to a 15-30% increase in revenue by identifying and retaining high-value customers. The scale of data allows for precise segmentation and resource allocation, which can translate into significant cost savings in customer acquisition and retention efforts.
For smaller companies with $10 million in revenue, the impact can be even more immediate. With targeted marketing campaigns based on CLV predictions, these companies could see a 10-20% improvement in marketing ROI. This is critical when resources are limited, and focusing on the most profitable customers can be a game-changer.
Conclusion
Customer Lifetime Value prediction is a powerful tool that allows businesses to prioritize their most valuable customers and optimize marketing strategies for long-term success. By following a clear process—collecting data, building models, visualizing insights, and continuously optimizing—companies can realize significant ROI, whether they’re large enterprises or smaller businesses. Leveraging AI platforms like DataRobot and visualization tools like Tableau, businesses can not only predict CLV but also take actionable steps to drive growth and profitability. If you're looking to dive deeper into executing a Customer Lifetime Value (CLV) prediction strategy for your business, consider taking a detailed course on AspinAI.com. This course provides a comprehensive guide on the entire process—from data collection to model building and optimization—using AI platforms like DataRobot. You'll learn step-by-step how to implement CLV prediction, create powerful dashboards with tools like Tableau, and maximize ROI. Whether you're managing a billion-dollar enterprise or a smaller company, the course equips you with the skills and insights needed to succeed. Check out the course at AspinAI.com.
Comments