Maximizing Security and Minimizing Loss: Implementing Real-Time Fraud Detection for Business Protection
- Steven Tedjamulia
- Sep 23, 2024
- 3 min read
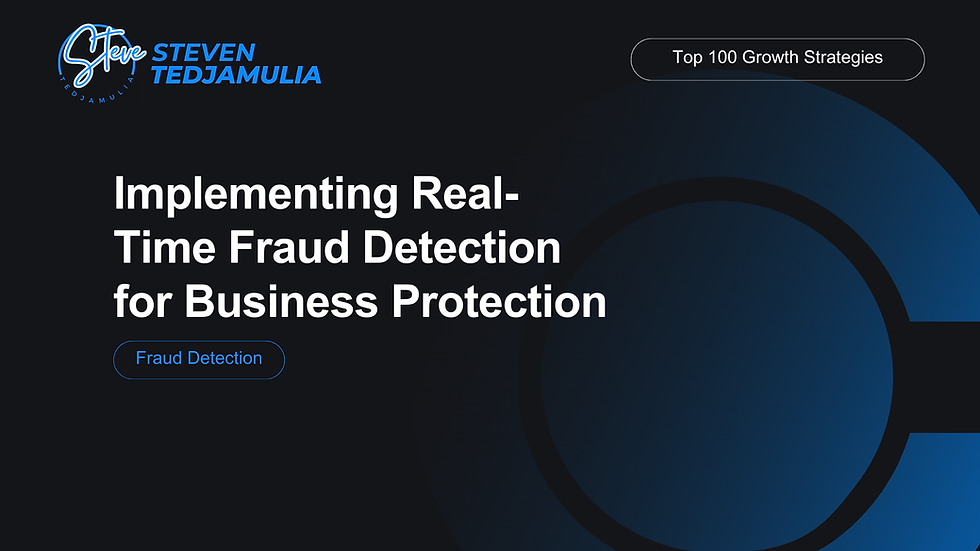
Maximizing Security and Minimizing Loss: Implementing Real-Time Fraud Detection for Business Protection - Fraudulent transactions are a significant concern for businesses, particularly in industries that handle large volumes of financial transactions or sensitive customer data. Implementing an AI-based fraud detection system can prevent substantial financial losses and protect your business's reputation. In this article, we’ll walk through the steps, time, resources, and predicted returns to help you successfully implement a real-time fraud detection system.
Step 1: Understand the Scope of Fraud
Time Required: 1-2 weeks
Resources Needed: Data analysts, fraud detection experts, financial risk team
Begin by analyzing the current scope and frequency of fraud within your business. Review historical transaction data, past fraud incidents, and customer behavior patterns to understand where vulnerabilities exist.
Actions:
Collaborate with the financial and risk management teams to review transaction records.
Identify fraud patterns and analyze common sources (geographies, transaction sizes, types of purchases, etc.).
Assess the current fraud detection processes and gaps.
Goal: Establish a baseline of current fraud activity, which helps in defining the objectives for the fraud detection system.
Step 2: Gather and Organize Relevant Data
Time Required: 2-3 weeks
Resources Needed: Data engineers, IT team, cloud storage solution
Collect and structure all relevant data necessary for fraud detection. This includes transaction data, user behavior data, and IP address data. Each of these datasets offers critical insights into identifying suspicious behavior.
Actions:
Set up pipelines to regularly collect transaction data (amount, frequency, geolocation, etc.).
Track and store user behavior data (logins, device usage, IP address changes).
Organize data in a centralized system (CRM, SQL database, or data warehouse) for easy access.
Goal: Build a robust and unified database that feeds into the AI-driven fraud detection tool.
Step 3: Choose and Implement AI-Driven Fraud Detection Tools
Time Required: 1-2 months
Resources Needed: AI/ML engineers, fraud detection tools (e.g., Sift, Signifyd), integration support
Choose a fraud detection tool that uses AI to monitor and analyze transactions in real-time. These tools continuously learn from new data and update their algorithms to identify suspicious behavior before fraud occurs.
Actions:
Research fraud detection tools like Sift and Signifyd to find one that fits your business model.
Work with AI/ML engineers to integrate the tool with your CRM and data systems.
Customize rules and risk thresholds to balance fraud detection and legitimate user experience (false positives should be minimized).
Goal: Have a real-time fraud detection system in place that automatically flags suspicious transactions.
Step 4: Test and Optimize the Fraud Detection System
Time Required: 1-2 months of testing and continuous optimization
Resources Needed: Data analysts, AI engineers, fraud risk managers
Once integrated, test the system by running historical data through the fraud detection tool. Adjust risk thresholds and algorithms based on test results to optimize detection accuracy.
Actions:
Perform a retrospective analysis using past data to measure the detection tool's accuracy.
Use real-time data to conduct live testing, flagging fraudulent transactions.
Collaborate with fraud risk managers to fine-tune detection sensitivity and reduce false positives.
Goal: Optimize the tool for maximum fraud detection with minimal disruption to legitimate transactions.
Step 5: Implement Monitoring and Continuous Learning
Time Required: Ongoing
Resources Needed: Fraud prevention team, AI engineers
AI-driven fraud detection systems thrive on continuous learning from both legitimate and fraudulent transactions. Set up ongoing monitoring and feedback loops to refine the tool’s performance over time.
Actions:
Develop a dashboard for real-time monitoring of flagged transactions.
Establish a system where fraud analysts review flagged cases and provide feedback to the AI tool.
Set up periodic performance reviews to ensure the system’s efficacy evolves alongside changing fraud tactics.
Goal: Create a self-improving system that continually updates to protect against new fraud schemes.
Predictive Returns and Business Impact
Predicted Time to ROI: 6-12 months
Investment: $50,000 - $150,000 for AI tool, integrations, and team resources
Annual Fraud Prevention Savings: Up to $1 million or more (depending on transaction volume and historical fraud losses)
Other Benefits:
Enhanced customer trust due to improved security
Reduced financial losses from fraud-related chargebacks and refunds
Minimized manual fraud review workload
Conclusion, Implementing Real-Time Fraud Detection for Business Protection
Implementing an AI-based fraud detection system requires an initial investment in time, resources, and technology, but the long-term benefits far outweigh the costs. By protecting your business from fraudulent transactions in real-time, you not only secure your financials but also build trust with your customers. Use this guide as a blueprint to build a robust fraud detection strategy and future-proof your business against ever-evolving fraud tactics.
For those looking to dive deeper into implementing AI-driven fraud detection and learn step-by-step how to execute this use case, AspinAI offers a comprehensive course. This course covers everything from data preparation and AI tool integration to optimizing your fraud detection system for real-world application. With detailed guidance from industry experts, you'll gain the practical knowledge needed to protect your business from fraud. To learn more and get started, visit AspinAI's Fraud Detection Course.
Comments