How Predictive Lead Scoring Can Maximize Conversions
- Steven Tedjamulia
- Sep 23, 2024
- 4 min read
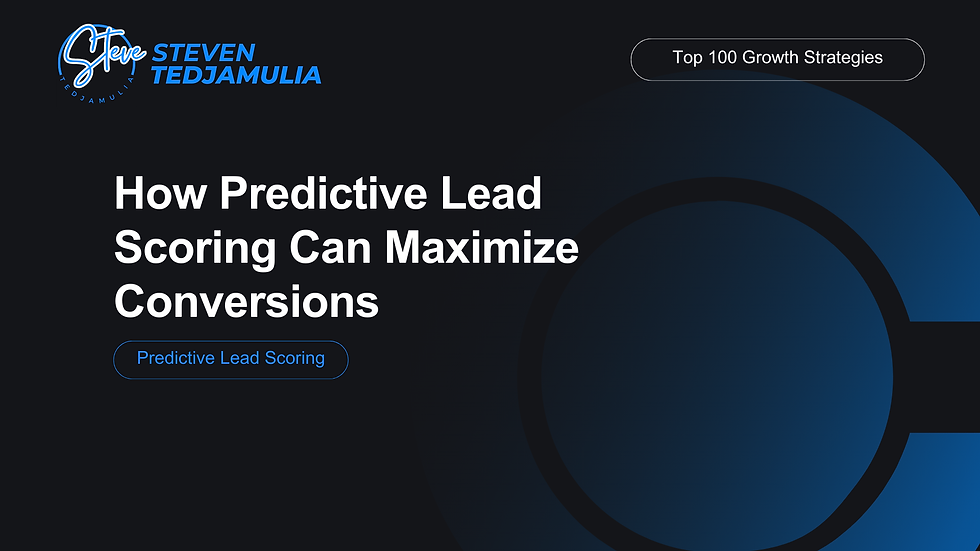
Predictive lead scoring is a powerful tool for sales teams to identify and prioritize high-potential leads, maximizing conversion rates and driving revenue growth. This approach leverages customer data and AI-driven insights to rank leads based on their likelihood to convert, allowing sales professionals to focus on prospects that offer the highest return on investment. In this article, we’ll outline the steps, resources, tools, and predictive returns of implementing a successful predictive lead scoring system.
Step-by-Step Implementation
Define the Objective
Goal: Rank potential leads based on their likelihood to convert.
Timeline: 1 week to finalize objectives and outcomes.
Resources: Sales and marketing teams, CRM managers, data scientists.
Collect and Prepare Data
Data Sources: Gather customer behavior data (website visits, product interactions), CRM data (past interactions, deals), and engagement data (email clicks, social media engagement).
Tools: CRM software like Salesforce, SQL for data extraction, Google Analytics, and other engagement tracking tools.
Timeline: 2-3 weeks for data collection and preparation.
Resources: CRM team, data analysts, data engineers.
Data Cleaning and Preprocessing
Task: Ensure data is clean, complete, and accurate by removing duplicates, filling in missing values, and normalizing data formats.
Tools: SQL, Python, or other data cleaning tools like Talend.
Timeline: 1-2 weeks for cleaning and preprocessing.
Resources: Data scientists, data engineers.
Feature Selection
Task: Identify the key variables (features) that impact lead conversion, such as engagement levels, demographic data, and previous purchase behaviors.
Tools: SQL, Python for feature extraction, and CRM tools for customer segmentation.
Timeline: 1-2 weeks.
Resources: Data scientists, marketing analysts.
Model Development
Task: Develop a predictive lead scoring model using machine learning algorithms. The model will score leads based on the probability of conversion.
Tools: AI platforms like DataRobot for automated model building, Python (scikit-learn) for custom model development.
Timeline: 3-4 weeks for model development, training, and testing.
Resources: Data scientists, machine learning engineers.
Model Evaluation and Tuning
Task: Evaluate the model using test data and tune it to improve accuracy. Key metrics to assess include precision, recall, and overall accuracy of lead ranking.
Tools: DataRobot, Python, or similar platforms with built-in evaluation tools.
Timeline: 1-2 weeks for evaluation and tuning.
Resources: Data scientists, machine learning engineers.
Integration with CRM
Task: Integrate the predictive model with your CRM (e.g., Salesforce) to automatically score leads and prioritize them for the sales team.
Tools: Salesforce (CRM), API connectors, or third-party integration tools.
Timeline: 1-2 weeks for integration and testing.
Resources: CRM managers, software developers.
Ongoing Monitoring and Optimization
Task: Continuously monitor the model’s performance, adjust it based on new data, and retrain as needed. Optimization involves adjusting lead scoring thresholds and responding to changing customer behaviors.
Tools: DataRobot for automated updates, CRM for real-time feedback.
Timeline: Ongoing, with major reviews every 3-6 months.
Resources: Data scientists, CRM managers, sales teams.
Resources Required
Human Resources:
Data scientists (for model development and optimization).
CRM specialists (for data collection and integration).
Sales and marketing teams (for initial input and ongoing feedback).
Tools and Technologies:
CRM Software (Salesforce or HubSpot): Essential for lead tracking and integration.
AI/ML Platform (DataRobot): To build and deploy predictive models.
Data Analysis Tools (SQL, Python): For data extraction and feature selection.
Data:
Historical customer behavior data.
CRM data on past leads and sales.
Engagement metrics (email clicks, website visits, social media interactions).
Predictive Returns
Increased Conversion Rates: By focusing efforts on high-scoring leads, sales teams can expect to see a 10-20% increase in conversions over time.
Example: A company closing 200 deals per quarter with a 5% conversion rate could potentially increase this by 15%, closing an additional 30 deals, representing an increase of $150,000 in quarterly revenue (assuming $5,000 average deal size).
Improved Sales Efficiency: Prioritizing leads means sales teams can allocate time and resources more effectively, focusing on prospects that are more likely to convert. This leads to a 20-30% increase in productivity.
Higher Customer Lifetime Value (CLV): By identifying the highest-quality leads, companies can not only close more deals but also target customers with the highest potential for repeat business, leading to a 10-15% increase in average customer lifetime value.
Faster Sales Cycles: Predictive lead scoring helps sales reps focus on the right leads earlier, reducing the time spent on unqualified prospects and potentially shortening sales cycles by 15-25%.
Cost Savings: By improving targeting, companies can reduce spending on ineffective marketing and sales outreach, leading to cost savings of 10-20% in marketing expenses.
Conclusion, Predictive Lead Scoring
Implementing predictive lead scoring can significantly enhance your sales process, helping you focus on high-quality leads that are most likely to convert. With the right data, tools, and approach, you can boost conversion rates, optimize resources, and ultimately drive revenue growth. By following the steps outlined above and using advanced AI tools like DataRobot, your organization can transform its lead generation efforts and maximize returns on your sales and marketing investments.
If you're interested in learning how to implement predictive lead scoring for your business in a step-by-step, hands-on manner, consider taking a detailed course on Aspinai.com. Their Predictive Lead Scoring course provides a comprehensive guide on gathering and analyzing customer data, building AI-powered lead scoring models, and integrating them into your CRM system. You'll learn the tools, techniques, and strategies to effectively rank leads and optimize your sales efforts for maximum impact. Visit Aspinai.com to explore the course and master predictive lead scoring today.
Comments