How AI-Driven Customer Service Automation Can Cut Costs and Improve Satisfaction
- Steven Tedjamulia
- Sep 23, 2024
- 4 min read
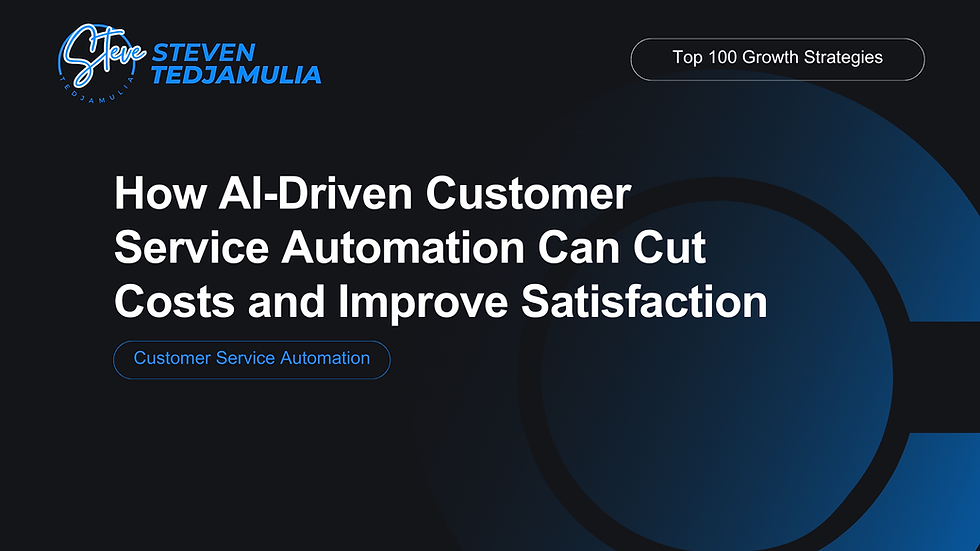
How AI-Driven Customer Service Automation Can Cut Costs and Improve Satisfaction - In today’s fast-paced business environment, customer service efficiency is paramount. AI-driven chatbots offer a powerful solution to streamline customer interactions, reduce response times, and cut support costs. By automating frequently asked questions and common customer service inquiries, businesses can significantly lower the burden on human agents while maintaining high levels of customer satisfaction.
In this article, we will break down the steps, time requirements, resources needed, and expected returns for implementing customer service automation using AI-driven chatbots.
Step 1: Define the Scope of Automation (1-2 weeks)
Tasks:
Gather Customer Service Data: Collect customer service interaction data from existing platforms. Focus on identifying recurring inquiries and frequently asked questions (FAQs).
Segment the Inquiries: Classify customer inquiries into categories—common queries, complex requests, and escalation points.
Set Automation Goals: Define the expected outcomes, such as reducing human interaction by 50%, cutting response times by 70%, or improving customer satisfaction scores by 15%.
Resources:
Access to customer interaction logs from existing tools (e.g., Zendesk, Intercom).
Customer service team for feedback on typical pain points.
Data analysts to identify patterns in the customer queries.
Predictive Returns:
Clear focus areas for automation.
Reduction in human agent involvement for repetitive tasks by 20% to 30%.
Step 2: Select and Configure Chatbot Platforms (2-3 weeks)
Tasks:
Choose a Chatbot Platform: Select from leading AI-powered platforms like Google Dialogflow, Zendesk, or Intercom that offer integration with existing customer service systems.
Customize Responses: Set up the chatbot to handle FAQs, using natural language processing (NLP) capabilities to ensure accurate responses to user queries.
Test NLP Accuracy: Conduct preliminary testing to evaluate how well the chatbot understands various customer inputs and refines responses for better accuracy.
Resources:
Customer service software (Intercom, Zendesk, etc.).
AI chatbot development platform (Google Dialogflow).
IT or customer service team for platform integration and setup.
Predictive Returns:
Reduction in response time for common inquiries by up to 50%.
The chatbot can handle up to 60%-70% of repetitive queries after the initial configuration.
Step 3: Train the Chatbot and Optimize the User Experience (4-6 weeks)
Tasks:
Data-Driven Training: Use the customer service data from step one to train the chatbot, refining its understanding of typical queries and responses.
User Interface (UI) Design: Ensure the chatbot is accessible on the most common customer touchpoints (e.g., website, app, social media channels).
Feedback Loop: Establish a process where unsatisfactory or complex inquiries are routed to a human agent, ensuring customers still get timely responses to unresolved issues.
Resources:
Machine learning specialists or chatbot consultants for refining AI training.
Customer service team for continuous monitoring and real-time feedback.
Front-end developers to ensure smooth integration across multiple channels (web, mobile, etc.).
Predictive Returns:
40% reduction in human agent workload as the chatbot successfully resolves common queries.
Improved customer satisfaction scores due to faster response times.
Step 4: Deploy, Test, and Scale (2-4 weeks)
Tasks:
Deploy the Chatbot: Release the chatbot in a live environment and monitor its performance closely during the first few weeks.
Test and Iterate: Gather customer feedback and refine the chatbot's performance. Ensure there are no major service disruptions and that customer queries are appropriately routed to human agents when necessary.
Scale Operations: Once optimized, scale chatbot operations to cover more complex queries, continuously training the AI to handle new scenarios.
Resources:
IT team for ongoing performance monitoring and technical adjustments.
Customer feedback tools to gather insights on chatbot effectiveness.
Regular involvement from the customer service team to monitor for issues.
Predictive Returns:
Up to 60%-70% of inquiries resolved through the chatbot.
30%-50% reduction in customer service operational costs.
Significant improvements in scalability, allowing the company to manage higher volumes of inquiries without proportional increases in staffing costs.
Step 5: Measure and Report Success (Ongoing)
Tasks:
Monitor Key Performance Indicators (KPIs): Track metrics like response time reduction, cost savings, and customer satisfaction (CSAT) scores.
Adjust as Necessary: Based on the results, adjust chatbot configurations, retrain the AI, or expand its capabilities to handle more complex requests.
Report Predictive Returns: Regularly report the ROI of the chatbot to stakeholders, emphasizing improvements in efficiency, customer satisfaction, and reduced labor costs.
Resources:
Data analysts for performance tracking.
Customer service managers to align chatbot goals with business objectives.
Predictive Returns:
Long-term support cost savings of 50%-60%.
Reduction in customer complaints related to slow response times.
Better resource allocation, allowing human agents to focus on high-impact tasks.
Conclusion: How AI-Driven Customer Service Automation Can Cut Costs and Improve Satisfaction
Automating customer service inquiries using AI-driven chatbots is a strategic investment that offers impressive returns in cost savings, customer satisfaction, and operational efficiency. By following the steps outlined, businesses can reduce their support costs, improve response times, and offer a seamless customer experience without overwhelming their customer service teams.
With the right tools, data, and strategic approach, companies can automate up to 70% of customer inquiries, leaving human agents free to focus on more complex and valuable interactions.
To dive deeper into the process of automating customer service with AI and learn how to execute this use case step-by-step, consider taking the comprehensive course available on Aspinai.com. The course, available here, covers everything from gathering customer interaction data to selecting and configuring AI-driven chatbot platforms, and it provides advanced insights on optimization and scaling for maximum returns. Whether you're new to AI or looking to refine your existing automation strategy, this course will equip you with the knowledge and tools needed to succeed.
Comments