Boosting Profitability with Inventory Demand Forecasting
- Steven Tedjamulia
- Sep 23, 2024
- 5 min read
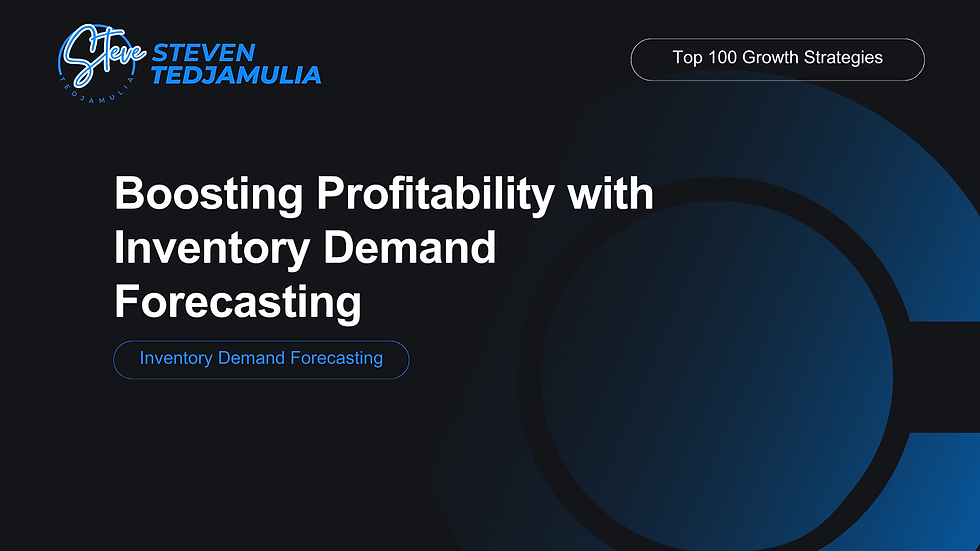
Inventory demand forecasting is a game-changer for businesses of all sizes. By predicting inventory needs, companies can avoid costly stockouts, prevent excess inventory, and optimize their supply chains. This use case is particularly valuable in today's fast-paced, data-driven business environment where effective inventory management directly impacts profitability. With advancements in machine learning and powerful tools like AWS SageMaker, Google AI, SQL, and Tableau, even small businesses can harness predictive analytics to streamline their operations.
For large companies generating billions of dollars in revenue, optimized inventory demand forecasting can save millions by reducing overstock and understock issues, improving cash flow, and increasing customer satisfaction. For smaller companies with revenues of around $10 million, the same approach can lead to significant gains in operational efficiency and margin improvements.
This article outlines the steps, time required, resources needed, and predicted returns for implementing inventory demand forecasting. By the end, you’ll have a clear roadmap for executing this strategy, no matter the size of your company.
Summary of Steps
Gather Historical Data: Compile sales data, seasonal trends, and marketing information.
Data Preparation: Clean and format data for use in machine learning models.
Select the Right Tools: Use AWS SageMaker for predictive models, SQL for data manipulation, and Tableau for dashboard visualization.
Develop Predictive Models: Train machine learning models to predict demand based on historical data.
Create Dashboards: Visualize demand forecasts using Tableau, showing inventory levels, predicted demand, and recommended stock levels.
Monitor and Adjust: Continuously update models and dashboards with new data to improve accuracy.
Step-by-Step Guide to Inventory Demand Forecasting
1. Gather Historical Data (Time: 1-2 weeks)
Data Sources: Historical sales data (at least 2 years), seasonality trends, marketing campaign data, and external factors (like economic or social conditions).
Tools: SQL for data extraction.
Skills Needed: SQL expertise, knowledge of data warehousing.
Who Should Do It: A data analyst or a business intelligence (BI) team.
The first step is to gather as much relevant historical data as possible. For large companies, this could involve accessing data from multiple systems or departments, including ERP and CRM platforms. Small businesses might only need data from a point-of-sale system or inventory management software. SQL is crucial for querying large databases and pulling relevant data.
2. Data Preparation (Time: 2-4 weeks)
Tools: SQL, Python or R (for data cleaning).
Skills Needed: Data cleaning, transformation, and basic statistical analysis.
Who Should Do It: Data scientists or data engineers.
The next step is to clean and prepare the data. This involves removing duplicates, handling missing data, and creating time-series data sets. For smaller businesses, the process might be simpler, but for large enterprises, this can be more complex, involving multiple data sources and formats. Data preparation is critical because the quality of the input data directly affects the accuracy of the demand forecasts.
3. Select the Right Tools (Time: Ongoing)
Tools: AWS SageMaker, Google AI (for machine learning), SQL (for querying data), Tableau (for visualization).
Skills Needed: Familiarity with cloud-based machine learning platforms (e.g., AWS, Google AI), basic SQL for data manipulation, and Tableau for creating dashboards.
Who Should Do It: A data science team or an external AI consultant for large companies; a freelance data scientist for smaller businesses.
AWS SageMaker is a powerful tool that simplifies building, training, and deploying machine learning models. It can process your data, automatically build and tune a model, and generate demand forecasts. Google AI is another viable option for companies that already have their data on Google Cloud. SQL is used for handling data throughout the process, and Tableau is essential for creating easy-to-understand dashboards.
4. Develop Predictive Models (Time: 4-6 weeks)
Tools: AWS SageMaker (or Google AI).
Skills Needed: Knowledge of machine learning algorithms (e.g., time-series analysis, regression), and cloud platform experience.
Who Should Do It: Data scientists or AI engineers.
Once the data is prepared, you can train machine learning models using AWS SageMaker. For demand forecasting, models like ARIMA, Prophet, or deep learning algorithms such as LSTMs (Long Short-Term Memory networks) are typically used. These models analyze past sales patterns, seasonality, and external factors (like marketing campaigns) to predict future demand. SageMaker’s built-in tools allow for quick model deployment and optimization.
5. Create Dashboards (Time: 2-3 weeks)
Tools: Tableau.
Skills Needed: Data visualization, SQL, Tableau experience.
Who Should Do It: Data analysts or BI teams.
Dashboards are essential for interpreting the results of your demand forecasting model. Tableau is an excellent tool for this, as it allows you to visualize inventory levels, forecasted demand, and recommended stock levels. The dashboard should display key metrics like predicted sales for the next month/quarter, safety stock recommendations, and over/understock risks. These visuals help decision-makers quickly understand and act on the insights generated by the forecasting models.
Dashboard Modules:
Inventory Levels: Current stock versus predicted demand.
Sales Forecast: Next month/quarter sales prediction based on seasonality and campaigns.
Safety Stock Alert: Highlight when inventory falls below recommended levels.
Overstock Risk: Alerts for potential overstock scenarios based on forecast data.
6. Monitor and Adjust (Time: Ongoing)
Tools: AWS SageMaker (to update models), SQL (to update data), Tableau (to refresh dashboards).
Skills Needed: Machine learning model monitoring, SQL, Tableau.
Who Should Do It: Data scientists and analysts.
As new data comes in (new sales data, updated marketing plans, etc.), the models need to be updated to remain accurate. AWS SageMaker allows for continuous model training with new data. Additionally, Tableau dashboards should be refreshed regularly to reflect the most current predictions and inventory levels.
Costs and Resources
For large enterprises, the initial setup cost might range from $100,000 to $500,000, depending on data complexity, size of data science teams, and cloud infrastructure. For smaller companies, costs might range from $10,000 to $50,000 if they use external consultants or pre-built machine learning models. Ongoing costs include cloud storage fees, data processing, and the time of data scientists or BI analysts.
Timeframe for Completion:
Large Company: 3-6 months.
Small Company: 2-4 months.
ROI and Predictive Returns
For a large company with annual revenue in the billions, inventory demand forecasting can save millions by reducing storage costs, improving cash flow, and boosting sales with fewer stockouts. Expected ROI could range from 5-10% in the first year.For a small company with $10 million in revenue, the ROI could be around 3-5%, as inventory optimization would free up capital and reduce losses from overstock or understock situations.
Skills and Who Should Be Involved
Large Companies: In-house data science teams, BI analysts, and cloud engineers. They will need experience with AWS SageMaker or Google AI, advanced SQL, and Tableau for visualization.
Smaller Companies: Freelance data scientists, AI consultants, or small data science teams with experience in machine learning, SQL, and Tableau.
Inventory demand forecasting is a powerful strategy that can transform how businesses manage their inventory, streamline operations, and increase profitability. Whether you’re a large enterprise or a smaller company, adopting this approach can lead to significant financial and operational gains.
For those looking to dive deeper into the implementation of inventory demand forecasting, Aspinai.com offers a comprehensive course that covers every step in detail. The course guides you through the entire process, from gathering and cleaning data to building predictive models using tools like AWS SageMaker and Tableau. You'll also learn how to create dynamic dashboards, optimize your forecasts, and monitor results in real-time. Whether you're a seasoned professional or just starting, this course provides the skills and knowledge you need to successfully execute this use case and drive impactful results. Enroll today at Aspinai.com to get started!
Commenti